Be Future Ready
With Digital Nest
Join us and accelerate your career
- 6246 + Students Trained
- 3000+ Career Transitions
- 23+ Courses Offered
- Classroom | Online | E-Learning
APPLY NOW
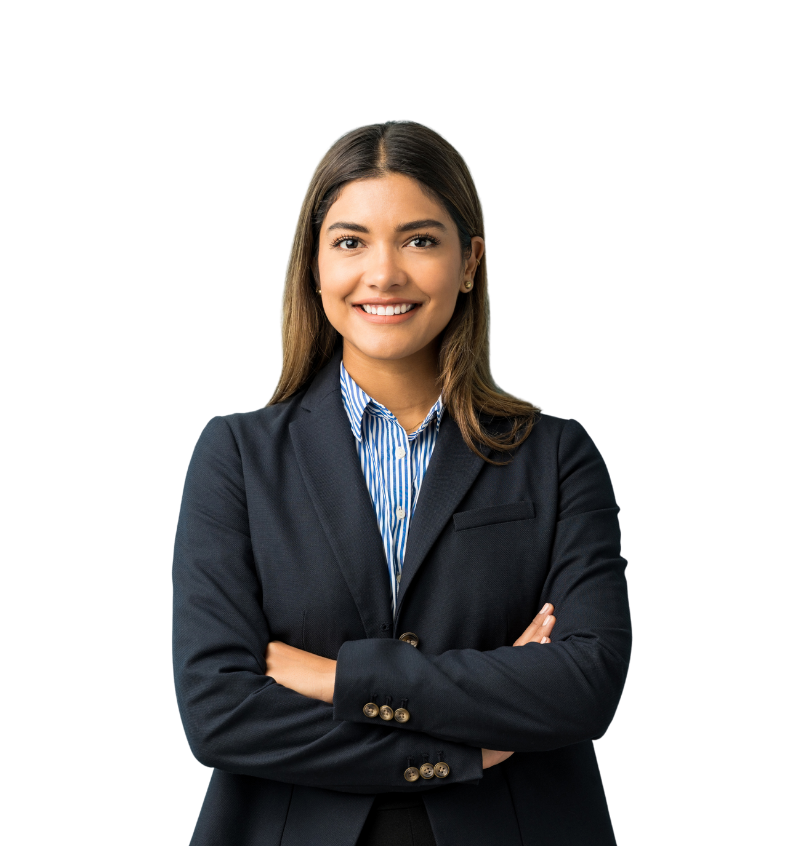
Be Future Ready
With Digital Nest
Join us and accelerate your career
- 6246 + Students Trained
- 3000+ Career Transitions
- 23+ Courses Offered
- Classroom | Online | E-Learning
APPLY NOW
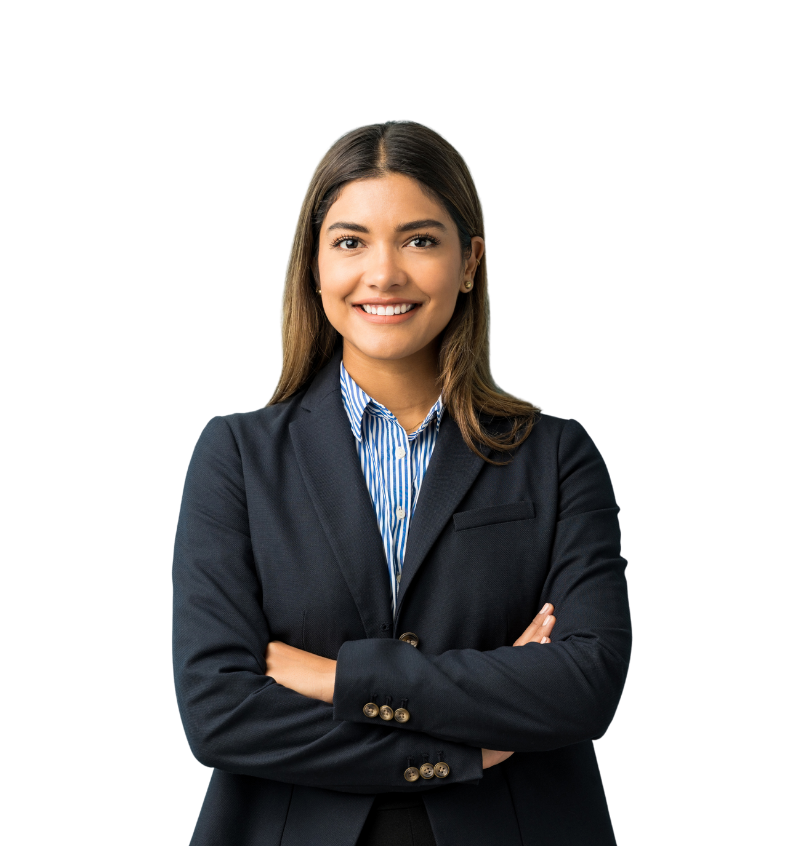
Our Collaborations with Industries and Academic Institutions

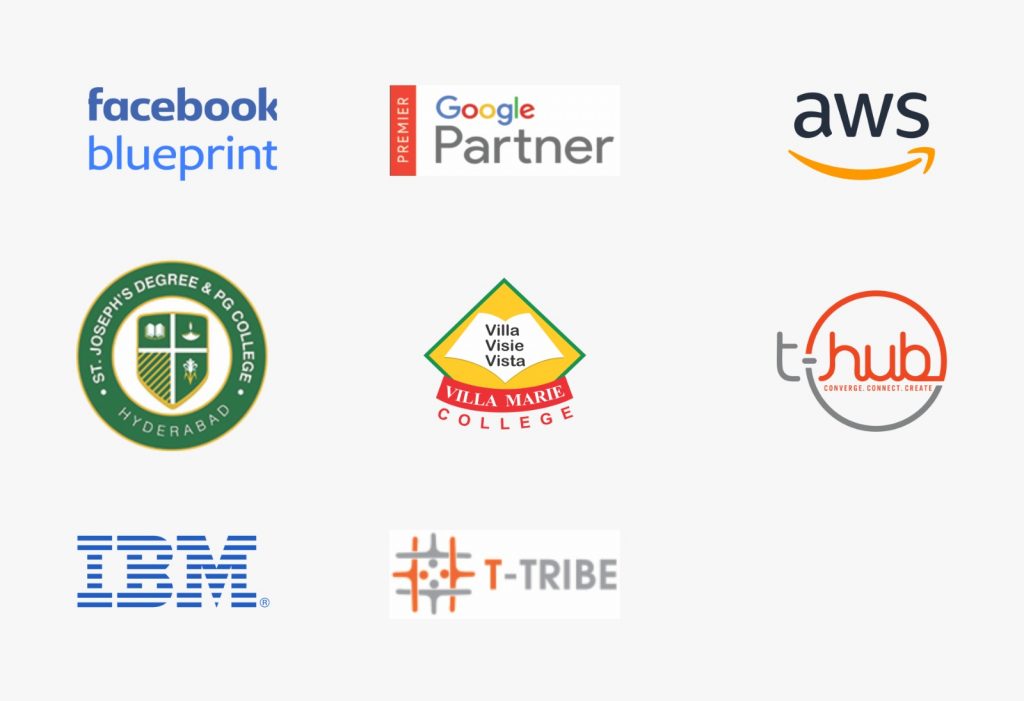
Explore Our Programs
For Students,Working Professionals, Freelancers & Business Owners
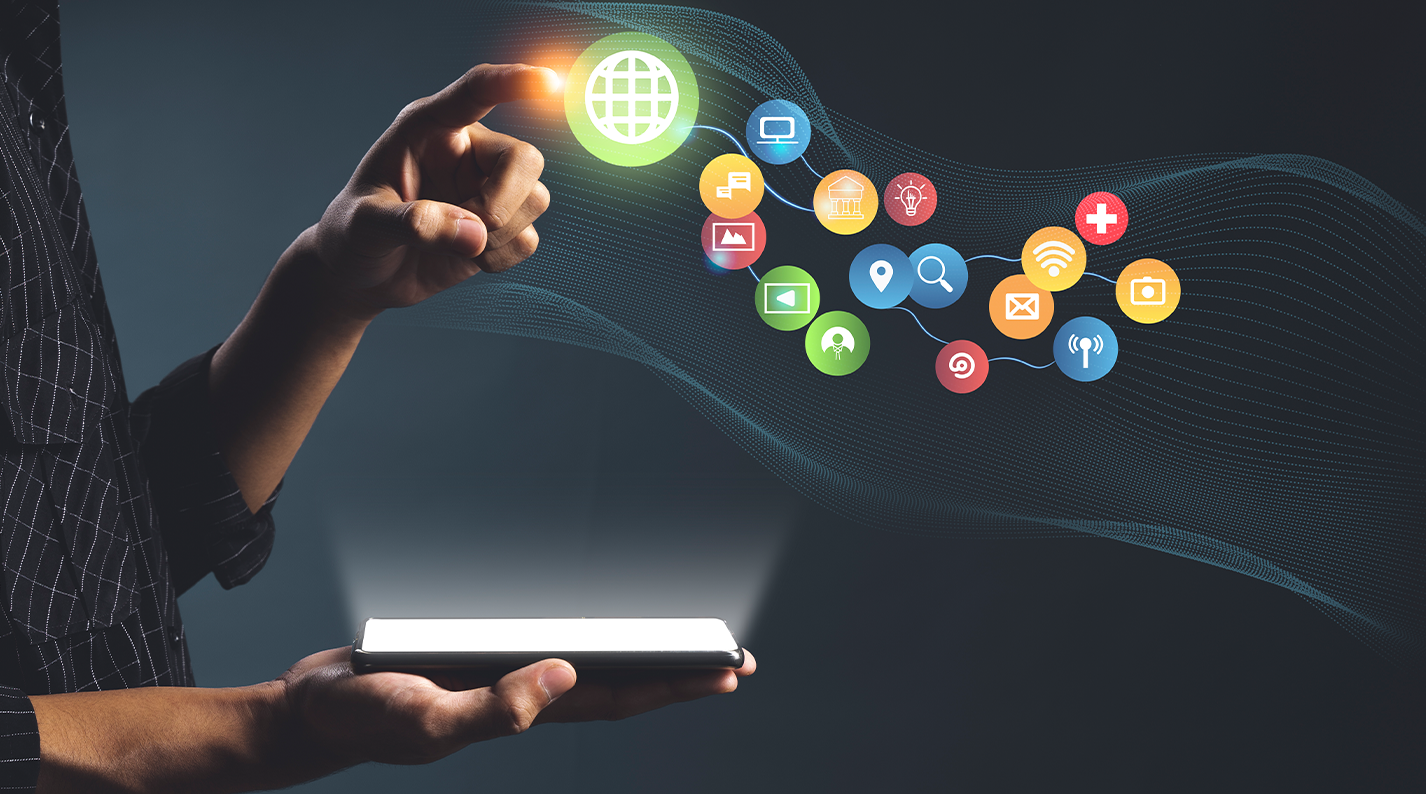
Post Graduate Program in Digital Marketing ( MBA Equivalent )
🗓️ 8 Months | Classroom Training
⏰ 320+ Learning Hours
👨💻 Assured Placements and Internships
📊 Live Case Studies and Projects
🎓 21+ Certificates
APPLY NOW
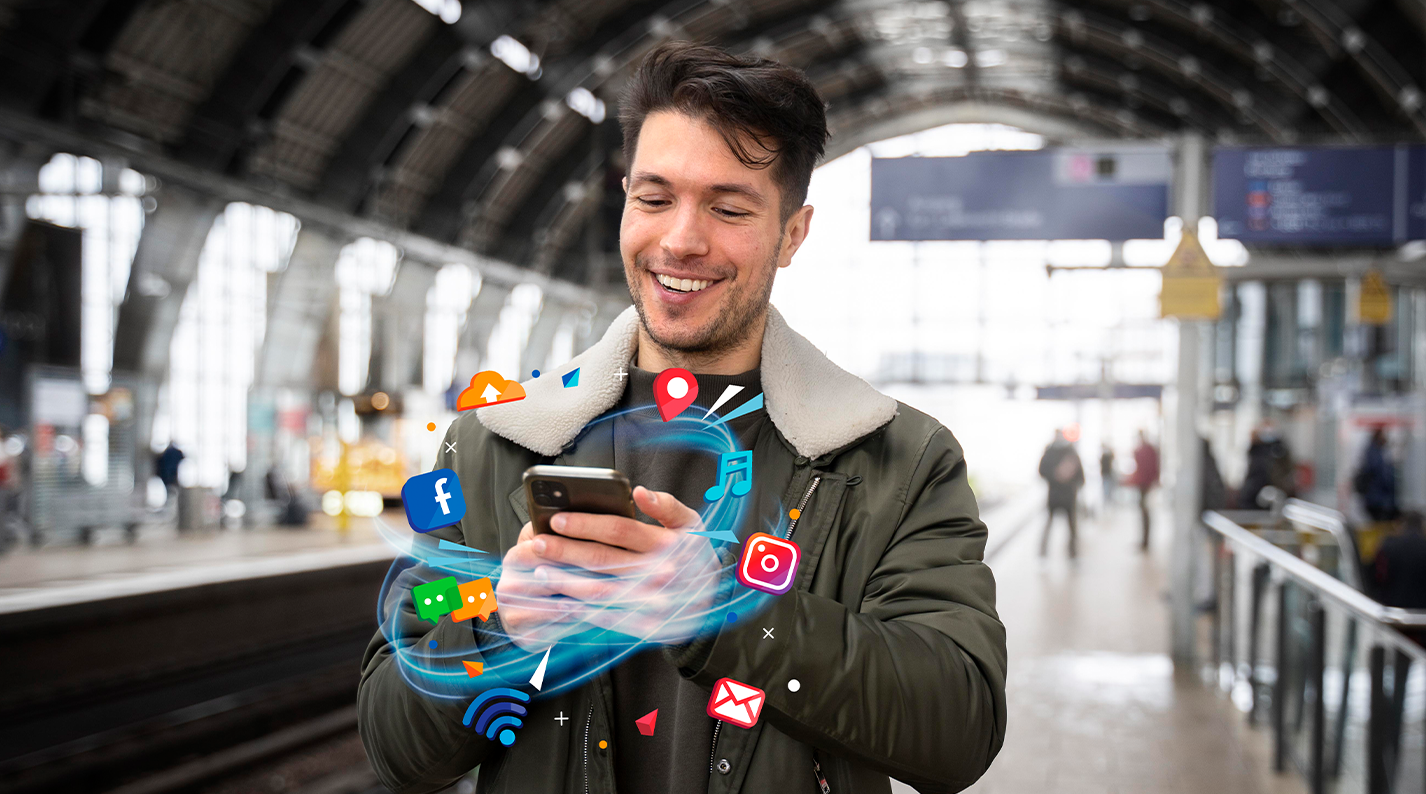
Certified Digital Marketing Program
Classroom | Online | E – Learning
🗓️ 5 Months
⏰ 200+ Learning Hours
👨💻 Assured Placements and Internships
📊 Live Case Studies and Projects
🎓 11+ Certificates
APPLY NOW

Digital Marketing Bootcamp
Classroom | Online | E – Learning
🗓️ 6 Weekedns
⏰ 55+ Learning Hours
👨💻 Placements assistance
📊 Live Case Studies and Projects
🎓 Certificate of Completion
APPLY NOW
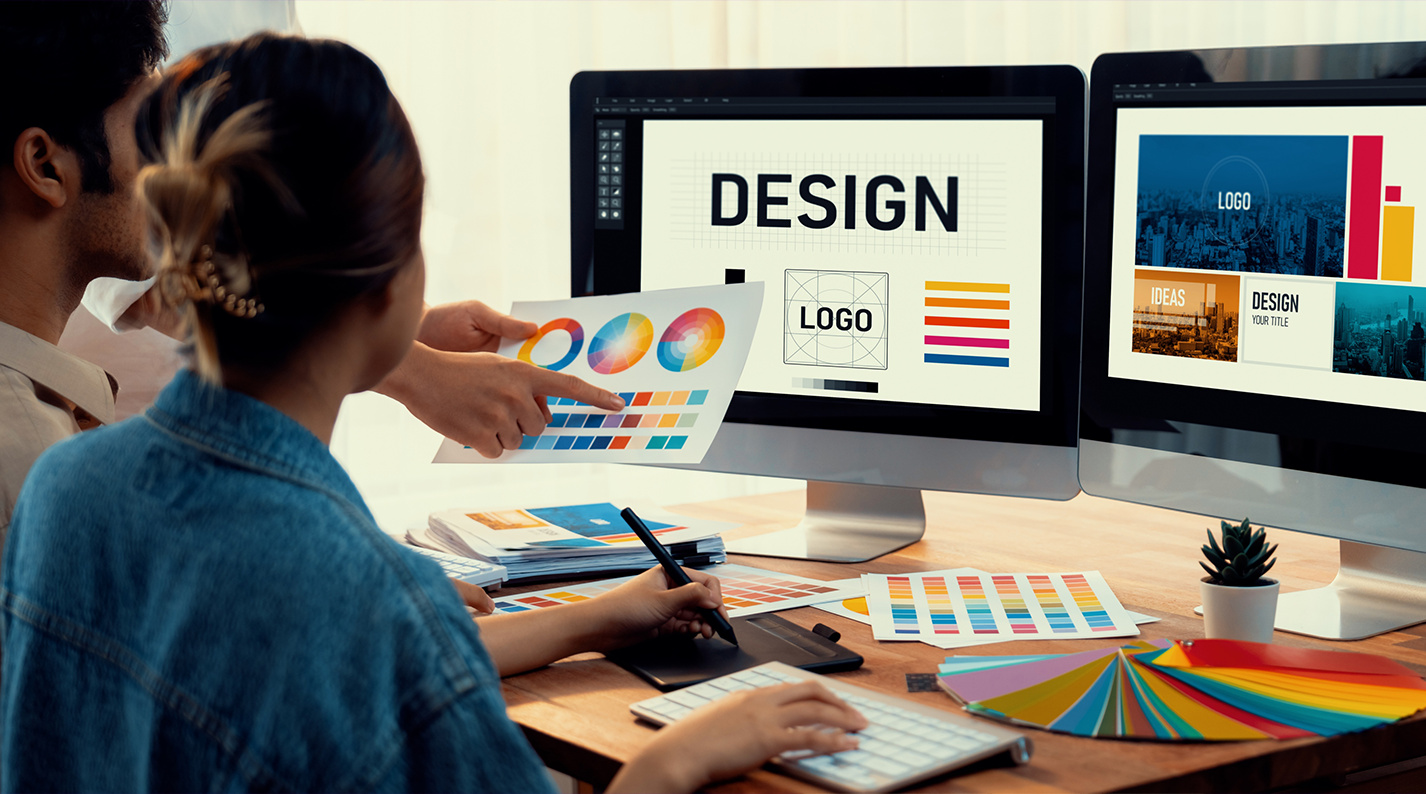
Graphic Designing
Classroom | Online | E – Learning
🗓️ 3 Months
⏰ 90+ Learning Hours
👨💻 Assured Placements and Internships
📊 Live Case Studies and Projects
🎓 Certificate of Completion
APPLY NOW
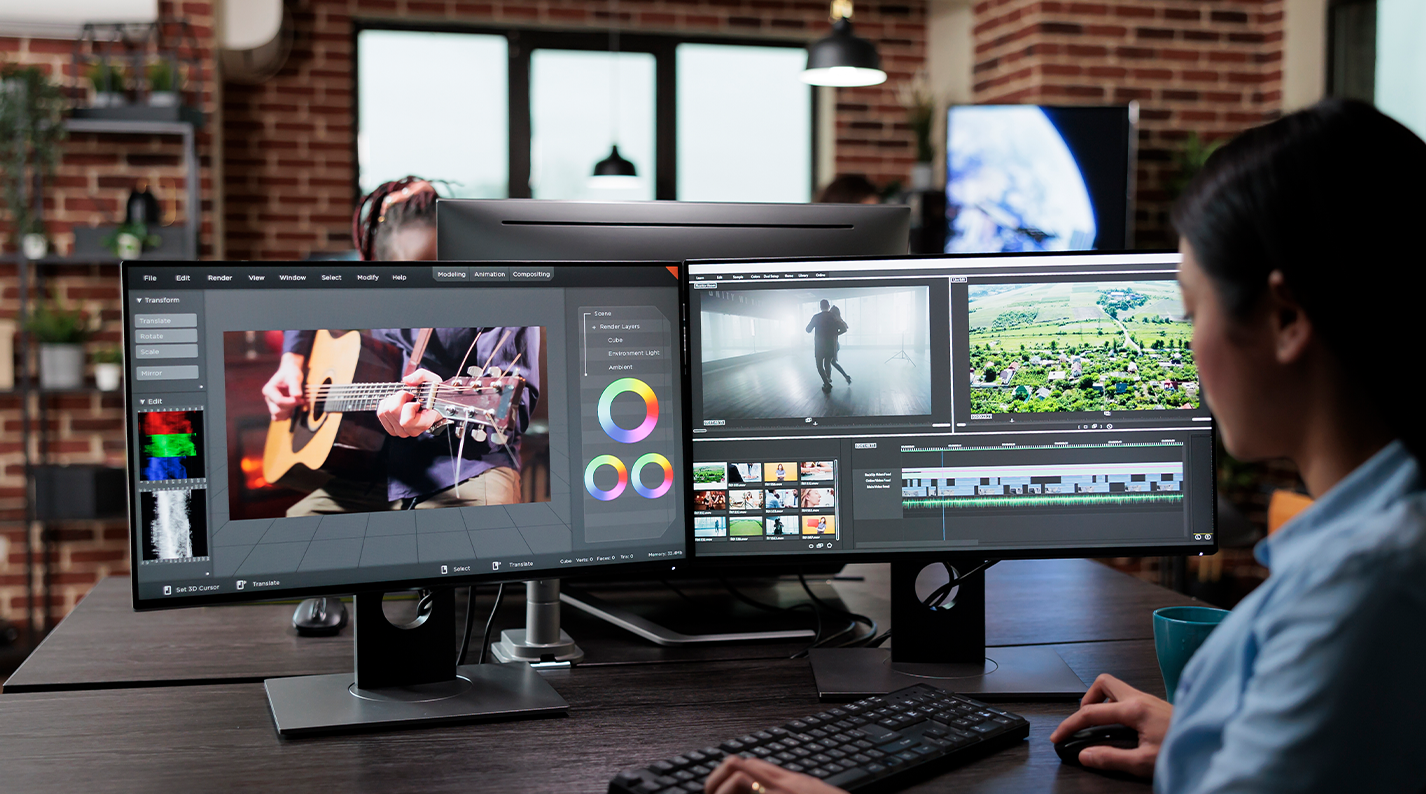
Motion Graphics & Video Editing
Classroom | Online | E – Learning
🗓️ 2 Months
⏰ 60+ Learning Hours
👨💻 Assured Placements and Internships
📊 Live Case Studies and Projects
🎓 Certificate of Completion
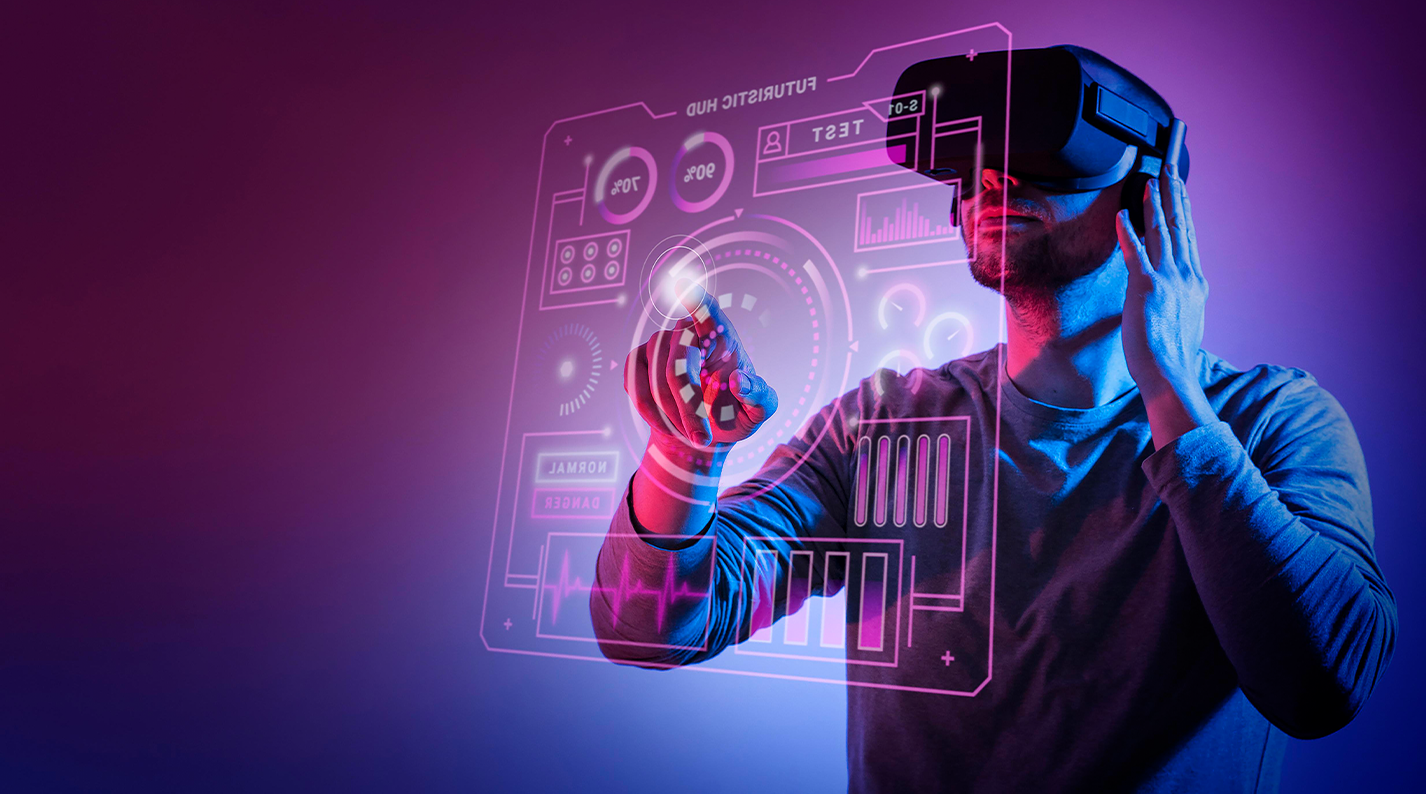
Post Graduate Program in Data Science
🗓️ 8 Months | Classroom Training
⏰ 100+ Learning Hours
👨💻 Assured Placements and Internships
📊 Live Case Studies and Projects
🎓 Certificate of Completion
Digital Nest Advantage
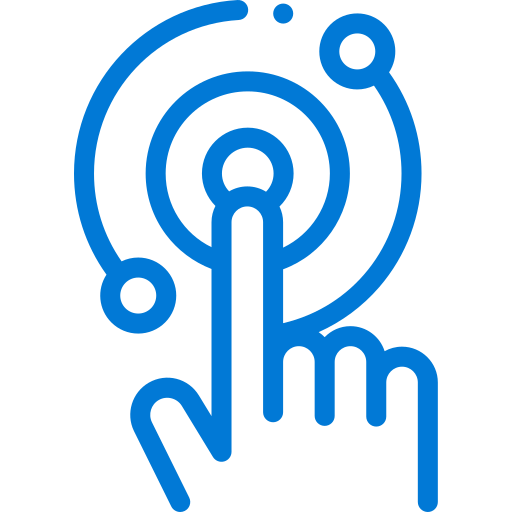
Interactive Learning
All the courses taught by us are interactive, with limited strength in a batch we make sure that you get the best experience

Learning Management System
Digital Nest has built a software for learners to learn using LMS where students can download material and freely access recorded sessions
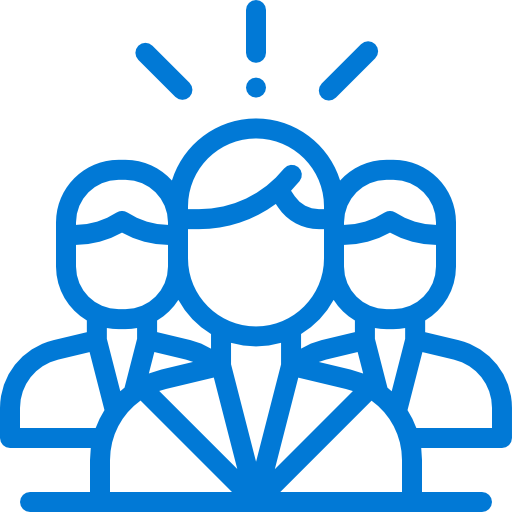
Network with Alumni
Digital Nest has over 6000+ alumnus working with various organizations. We have a networking app to connect with the alumni as well
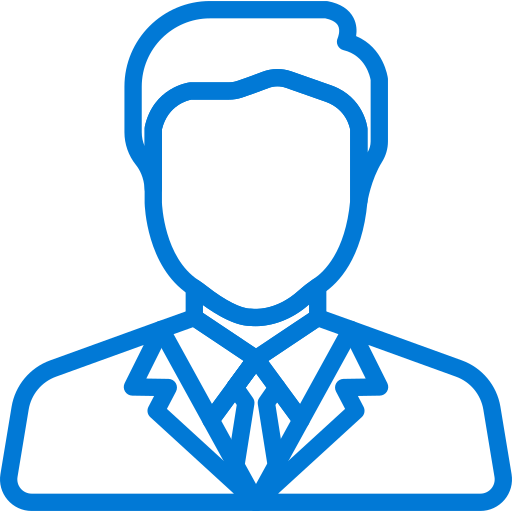
Dedicated Program Manager
We have a dedicated Program Manager who will constantly take feedback from the learners to have a better learning experience

Placement Support Team
We conduct mock interviews and screen CV’s to make you industry ready. We have a unique job portal to list the jobs posted by recruiters
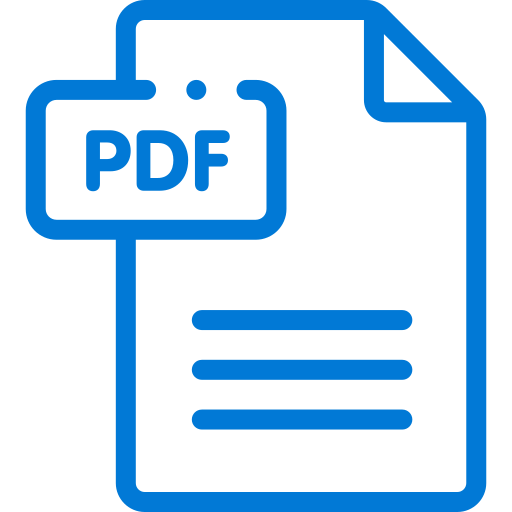
Case Studies & Assignments
All our courses are not just theoretical, we teach using practical methods such as simulation exercises, projects, assessments, etc
Digital Nest Advantage
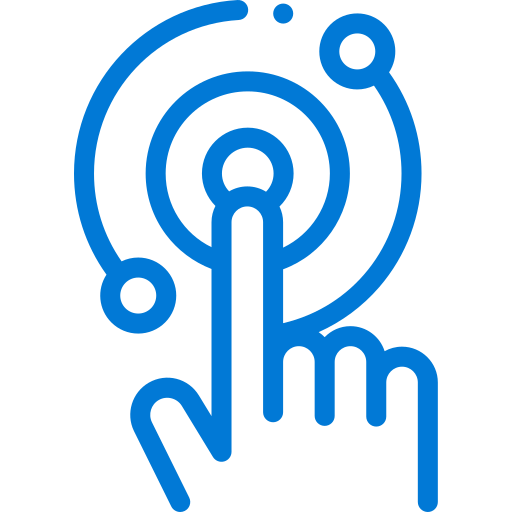
Interactive Learning
All the courses taught by us are interactive, with limited strength in a batch we make sure that you get the best experience

Learning Management System
Digital Nest has built a software for learners to learn using LMS where students can download material and freely access recorded sessions
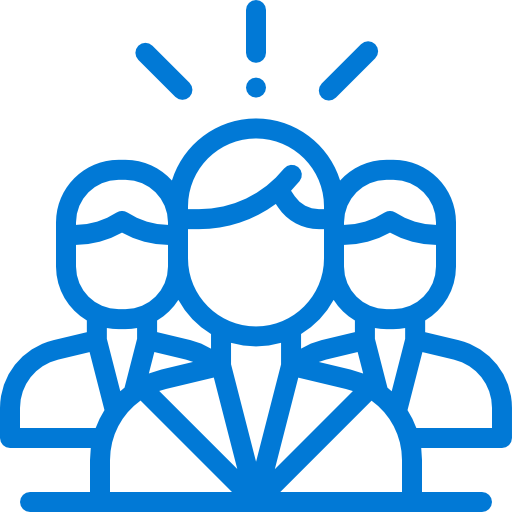
Network with Alumni
Digital Nest has over 6000+ alumnus working with various organizations. We have a networking app to connect with the alumni as well
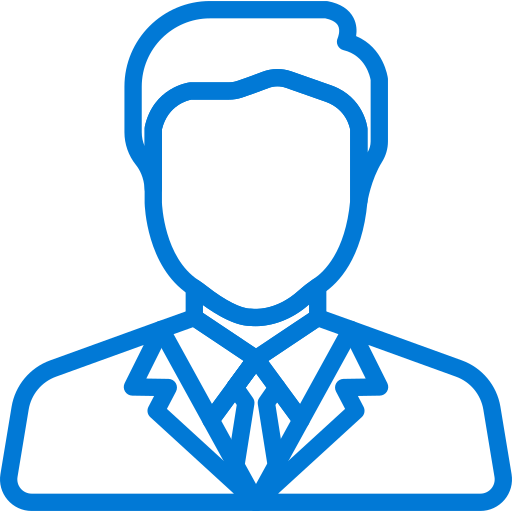
Dedicated Program Manager
We have a dedicated Program Manager who will constantly take feedback from the learners to have a better learning experience

Placement Support Team
We conduct mock interviews and screen CV’s to make you industry ready. We have a unique job portal to list the jobs posted by recruiters
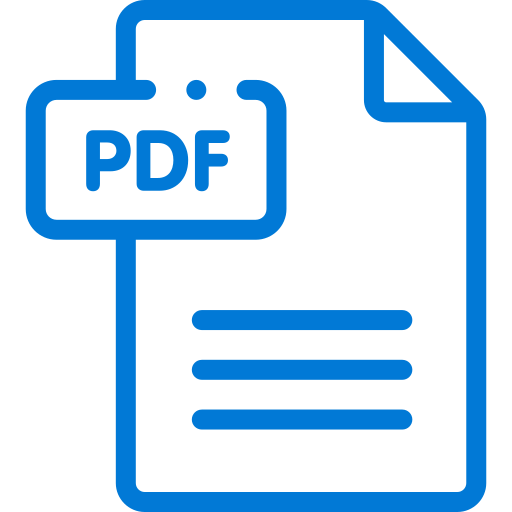
Case Studies & Assignments
All our courses are not just theoretical, we teach using practical methods such as simulation exercises, projects, assessments, etc
Awards & Recognition
Appreciation for the Excellence in Education
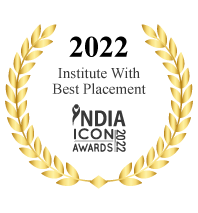
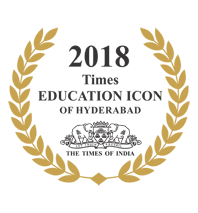
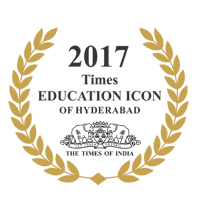
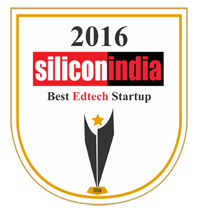
Partner With Us
Upskill your Employees or Students using our tailor made Industry relevant Courses
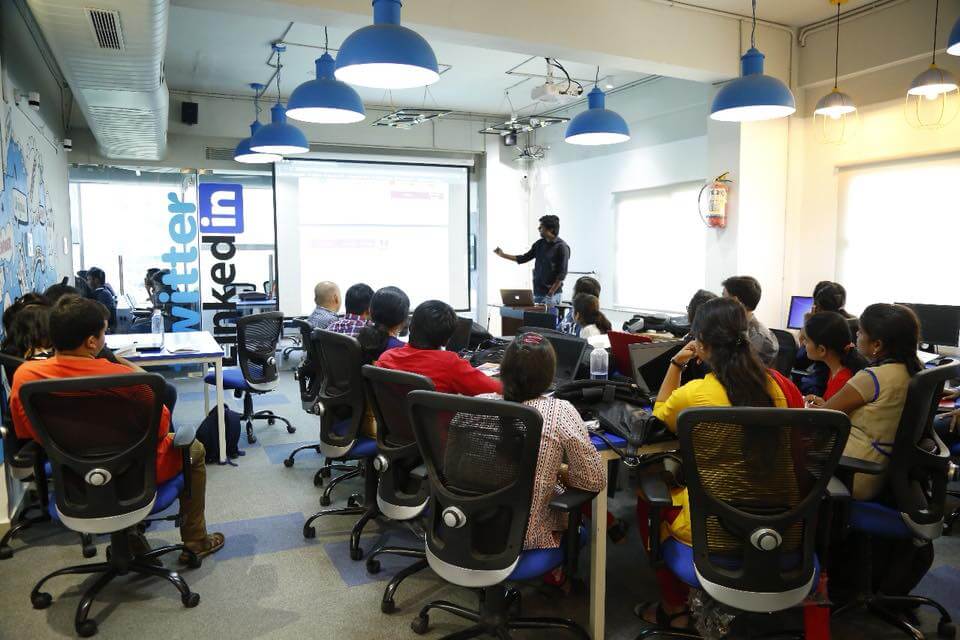
Training for Educational Institutions
Digital Nest has tailor made Industry relevant courses for Students to pursue and Excel along with their Academics , we deliver Seminars, Webinars, Workshops and short term Certificate courses based on the Requirement
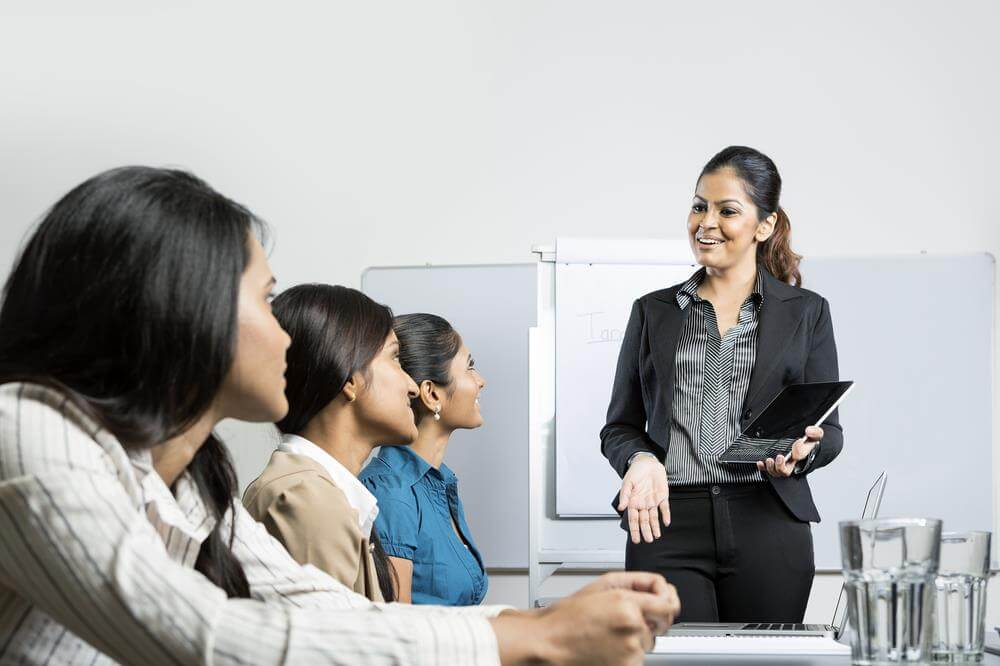
Employee Training Solutions For Corporates
Digital Nest Team is ready to provide cutting edge technology Courses for Corporates. We have real time expert trainers who are Proficient in handling Upskilling programs through Workshops .
APPLY NOW
Why Choose Digital Nest?
Our
Alumni Testimonials
Rated Avg 4.5* Out Of 5 by 2000+ Learners
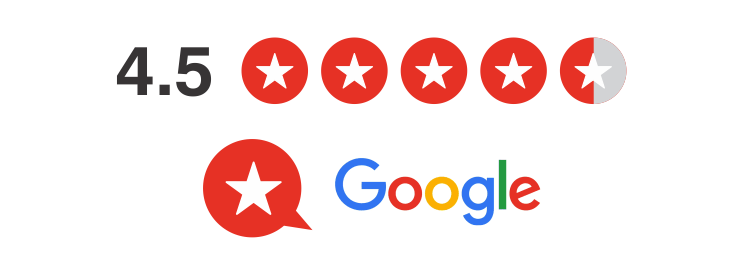
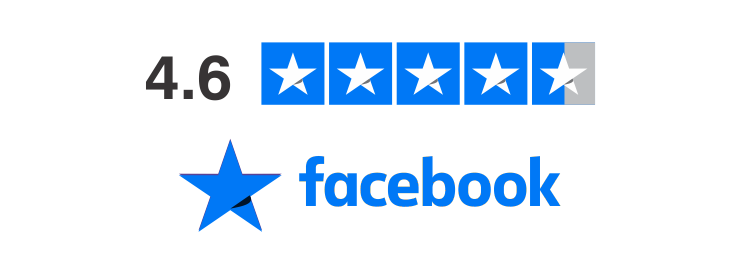
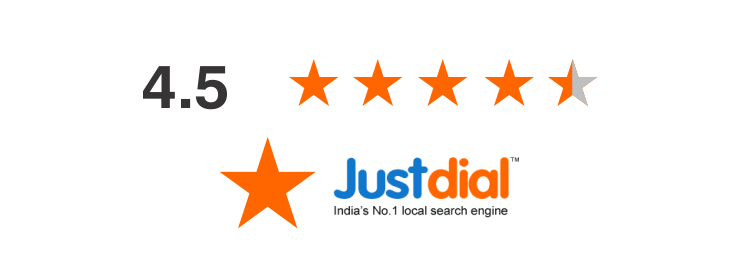
I was very satisfied with the Digital Marketing course in Digital Nest. The course was really practical and useful, especially the teaching practices where you could put what you have learned into practice while being observed by experienced tutors that guide you into the right path of your career
I have joined Aws course for 45 days! it as very good interactive program by Digital Nest in Hitech city branch. srikanth sir explained Aws concepts with real time examples. the most interesting part during the course was dealing with the projects, it was very challenging and thrilling
Digital Nest is one of the best institute for Python training. Trainer has in-depth knowledge about all the Python modules, the subject I gained I am sure I might have not gotten from any other institute, he gave more than 100+ coding examples. I highly recommend Digital Nest for Python training in Hyderabad
Though I come from non technical background, I never failed to ask doubts and participate in the assignments. The Digital Nest is a great platform, even for non-technical students or slow learners like me. I feel so grateful for Digital Nest and especially their Data Science trainers for instilling so much confidence in me. I owe it all to Digital Nest for what I’m now. they helped me grow, They have built a really good ambiance, and house a really good team of counselors that help you through the whole journey.
Our Learners Hail From
Students , Working Professionals & Business Owners From Various Start Ups And MNC’s
Admissions Support
Queries, Feedback & Assistance